
AI can already help clinicians make better decisions and either augment or even replace human judgments in specific areas of healthcare. Source: Shutterstock
AI is becoming the clinician’s new sidekick
THE next time you visit the local hospital for a chest X-ray, scan, or even a check-up, artificial intelligence (AI) could be having more of a role in your health than you might expect.
While we are many years away from a completely automated health service, AI can already help clinicians make better decisions and either augment or even replace human judgments in specific areas of healthcare.
Researchers at the John Radcliffe Hospital in the UK have developed an AI diagnostics system that is highly accurate in diagnosing heart disease around 80 percent of the time. At Harvard University, researchers have created a microscope that can detect potentially lethal blood infections.
Technologically-based systems help physicians by combing through the gigabytes of data available from journals and textbooks, as well as information from real-time clinical practices. Additionally, AI systems can elicit information from huge patient numbers in order to help predict health outcomes and improve ongoing wellness for an individual.
#Brain scan and artificial intelligence could predict whether OCD will improve with #treatment @PNASNews https://t.co/ixKl1BjOKY
— Medical Xpress (@medical_xpress) February 14, 2018
Clinical data starts with generalities like demographics and extends to electronic patient records, data from medical devices, doctors’ notes, reports on examinations, laboratory results and imagery; all this helps form a complete picture of the patient.
While the uses of data are not limited (once normalized and clean), currently AI research centers around three particular blights on the human condition: diseases of the nervous system, cardiovascular disease, and cancer. All three are leading causes of death, and all benefit from early diagnosis.
Two categories of AI
In healthcare, AI methods fall mainly into two camps. First is machine learning (ML), which analyzes structured data such as the genetic background of patients, images derived from radiology, and any electronic patient records which may exist.
ML procedures attempt to aggregate multiple patients’ traits in order to produce figures on the probability of outcomes for existing and new presentations.
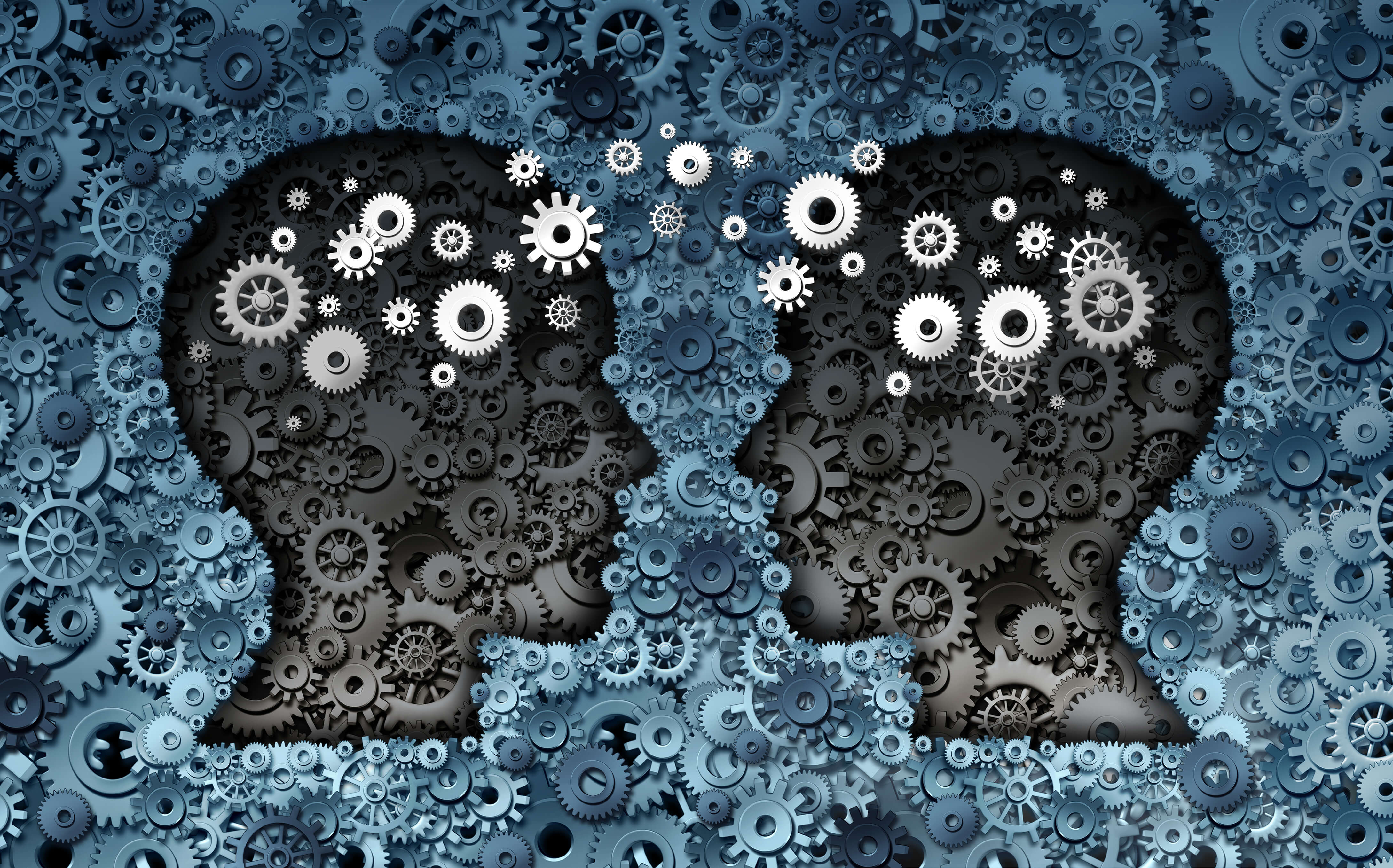
ML routines are good at processing large quantities of data very quickly. Source: Shutterstock
The second category of AI used in medical settings is natural language processing (NLP). It collates information from clinical notes, medical journals, publications, and otherwise unstructured data, in order to add to the overall picture of the patient. NLP processes text so it’s machine-readable data which can then, in turn, be fed into ML routines.
Therefore, in reverse order:
Natural language processing
Large amounts of clinical information can be found in text derived from doctors’ examinations (traditionally illegible to mere human eyes), lab reports, notes on surgeries, and discharge papers. These generally lack structure in themselves but also do not correlate with one another’s data fields. Therefore, in their raw state, these records are not suitable for algorithmic processing.
NLP, therefore, is highly useful at extracting useful information from these types of texts in order to assist clinical decision-making.
A natural language processing pipeline comprises of two parts: text processing, and classification of that processed text.
NLP-derived data has proved highly effective in reading chest x-ray reports, providing warnings that certain patients would need antibiotics in order to avoid infections. Plus, NLP has been effective in identifying symptoms of cerebral aneurysms when signs of this condition were spread across multiple, previously unaligned sources.
Machine learning
ML algorithms are efficient at processing patient data such as age, gender, disease history, baseline data, and the information as pre-prepared by NLP, as discussed above.
Additionally, systems can process diagnostic imaging, gene data, results from physical examinations, medication types and quantities, and so forth.
Algorithms in common use include discriminant analysis, logistic regression, neural networking (including deep learning – the use of multiple neural networks in tandem), and support vector machine routines.
ML routines are of course good at two things: processing large quantities of data very quickly and changing the way the data is processed according to learned lessons. Both of these abilities will make ML a key aspect of future medical care.
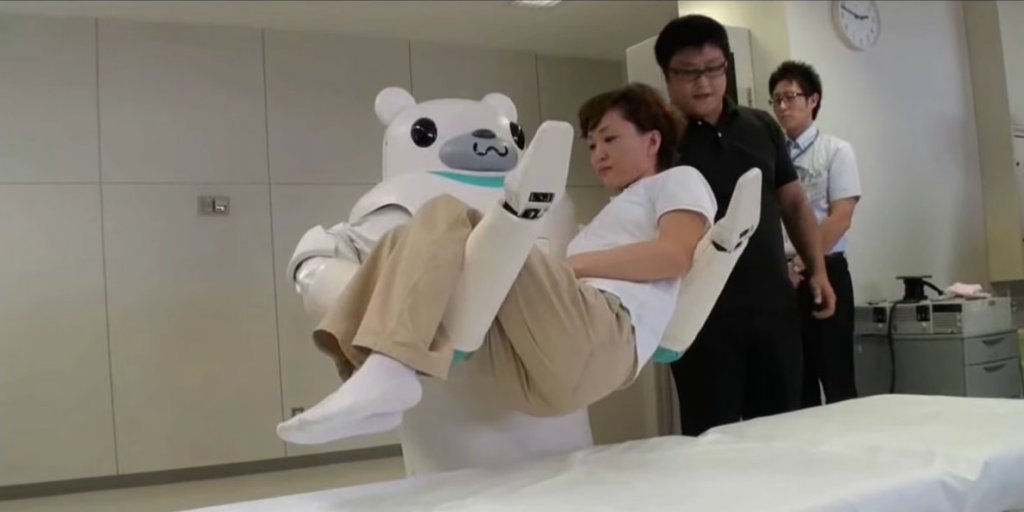
Technology could help an increasingly elderly APAC population when carers can’t. Source: YouTube/Plastic Pals
The cloud
For healthcare providers, there are several cloud-based AI powerhouses available ready- or partially-built. Primary amongst these is IBM’s Watson which offers both machine learning and natural language processing modules.
In clinical trials, 99 percent of treatment recommendations derived from Watson correspond with clinical decisions made in an oncological study.
Watson has already started to make an impact on actual clinical practices: for instance, by analyzing genetic data, the algorithms identified a rare secondary leukemia caused by myelodysplastic syndromes in Japan.
(Non-Medical) Problems
As is often the case, governments and legislative bodies lag behind the curve of technology. Current regulations (although differing across the globe) often limit the use of AI systems in clinical settings.
For instance, in the USA, the Federal Drug Administration guidance classifies AI systems to be only general wellness products, and are only permitted in areas considered to be low risk.
The second problem is one of exchange of data.
Artificial Intelligence can help make medical equipment smarter, imaging results faster and examinations more precise. #AI. https://t.co/o3QAdGhzve #digitalhealth pic.twitter.com/3GL1pnWaM3
— Siemens Healthineers (@SiemensHealth) February 14, 2018
AI systems need a continuous drip-feed of input from clinical studies and real-life practices. After an AI system is deployed following an initial training period, it is important that the supply of data continues, to both improve the computational system and patient outcomes.
Often, healthcare environments do not provide sufficient incentives for sharing data, plus of course, there are understandable objections raised to exchanging even anonymized data.
Conclusion
Many of the predictions about artificial intelligence contain dystopian outcomes, but in the field of medicine, the reality is more likely to be one of automata aiding and guiding their human clinician colleagues.
The days of the robot doctor may be many eons distant, but with the power of AI, human lives may well be extended and improved along the way.
Additional material from the BMJ.
READ MORE
- The new age in running an MSP in A/NZ & beyond
- Google parent Alphabet eyes HubSpot: A potential acquisition shaping the future of CRM
- Microsoft splits Teams from Office Suite; who benefits?
- Unveiling the reality of incognito mode from Google and online privacy
- How Bonzai is disrupting the digital ad landscape with rapid innovation