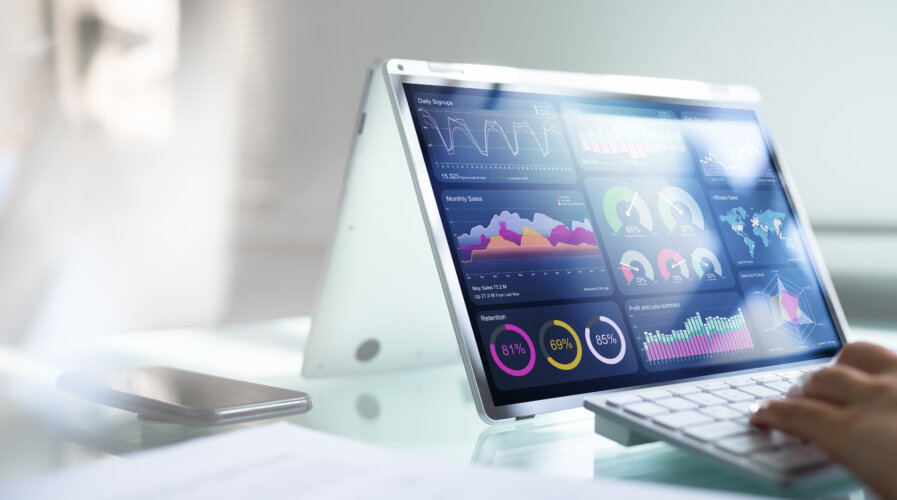
(Source – Shutterstock)
Achieve deeper business insights with a flexible hybrid data strategy across clouds
Article by Daniel Hand, APAC Field CTO at Cloudera
Organizations face numerous challenges when managing and gaining insight from data. As data is increasingly being created and stored in multiple locations, adopting a flexible hybrid data strategy becomes essential to managing and orchestrating disparate data sets.
While technology alone will not solve the challenges outlined below, it is a critical element of the solution that organizations must leverage in tandem with guiding principles and policies for dealing with them.
The first challenge focuses on supporting innovation and business agility. This includes how we can democratize access to data and data assets. This subsequently helps organizations make better use of the data they have and do so without having to unnecessarily go through data gatekeepers.
Another element of innovation is helping organizations quickly expand and provide Data Products and Services into new markets. If this requires establishing a new analytical cluster in a data center, especially in a new country, it often requires extended lead time. Managing data and running analytics in the public cloud can significantly accelerate the time to value, but risk and operational complexity need to be managed.
There are distinct differences between gaining insights in near real-time versus traditional batch analytical approaches. The value of insight from data decreases with age, so organizations increasingly need to augment batch analytics with near real-time stream processing.
Managing operational risks
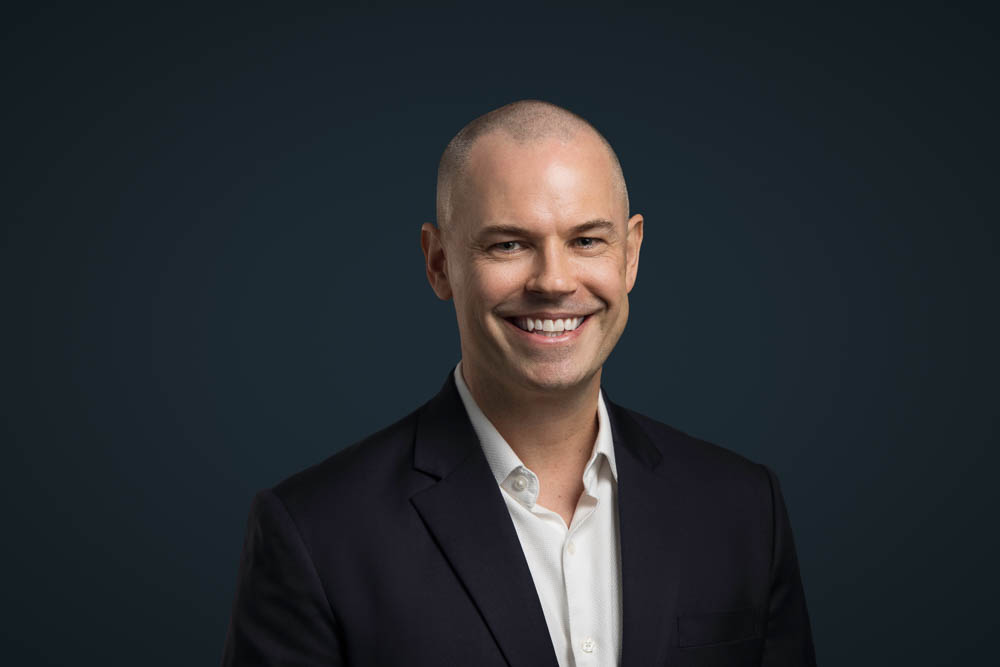
Daniel Hand, APAC Field CTO at Cloudera
The next challenge focuses on managing operational risk, which includes how organizations can ensure that security policies and controls are applied consistently and reliably across each supported environment.
Another variation on this is the need to capture data lineage and provenance across the entire data lifecycle. The value of insight derived from data is reduced if organizations lack visibility into where data comes from, and who or what has had access to it to transform it during its lifetime.
As the amount of data being captured increases exponentially, there is an increasing need to automatically profile data, classify it and apply suitable controls. For example, does a new data set contain sensitive Personally Identifiable Information (PII) data? There is also the associated challenge of how to efficiently manage and analyze data at a multi-petabyte scale.
Organizations also need to safely and efficiently move data and applications between environments, potentially in response to changes in regulation and governance. This isn’t just about potential repatriation from the cloud to on-premises, but also between public cloud providers if one platform is deemed to provide insufficient controls by a regulator in response to changes in policy.
Managing operational complexity
The last challenge focuses on managing the operational complexity of disparate data sets and analytical workloads. Adopting different solutions across each public cloud platform and on-premises will place a significant burden on operating expenses and maintaining a team equipped with the right skills. Collectively these factors will lead to and increase operating risk and impact agility.
Based on the three challenges presented earlier, here are some guiding principles and policies to help organizations overcome obstacles as they build a robust data strategy. When considering technology, organizations should keep in mind the benefits of supporting hybrid multi-cloud infrastructures, and open ecosystems of processing engines while providing options for adopting integrated sets of analytical services across the data lifecycle, and defining security policies and controls that can be consistently enforced across each supported environment.
Ideally, the technology should be scalable to support not only today’s data management and analysis needs but those that can be predicted within the next few years, and designed for the cloud to allow compute and storage to scale independently.
The hybrid data strategy
The ability to support modern data architectures such as data fabric, data lakehouse and data mesh continues to influence the solutions offered to enterprises today. A platform that is able to manage disparate data sets consistently across multiple environments combined with the unification of the data lake and data warehouse, together with supporting data as a product, domain ownership and self-service address the recommendations. The ability to do this consistently across the entire data lifecycle across public and private clouds supported by a shared security and governance fabric differentiates a hybrid data platform from other enterprise data platform solutions. This ability has also been integral to helping the world’s largest organizations envision and implement a flexible data strategy.
The views in this article are those of the author and may not reflect the views of this publication.
READ MORE
- Strategies for Democratizing GenAI
- The criticality of endpoint management in cybersecurity and operations
- Ethical AI: The renewed importance of safeguarding data and customer privacy in Generative AI applications
- How Japan balances AI-driven opportunities with cybersecurity needs
- Deploying SASE: Benchmarking your approach