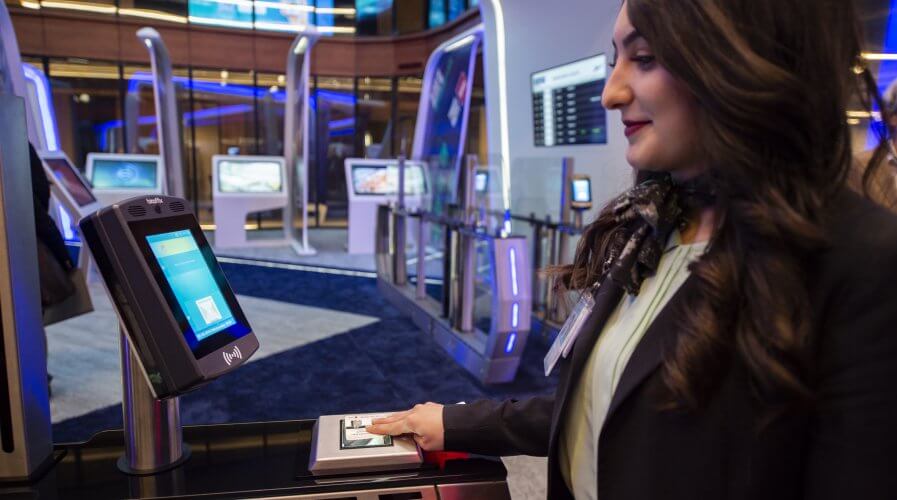
An attendant does the test of facial recognition using biometry technology in the “innovation hub” of Istanbul airport. Source: AFP
Ethical facial recognition? WEF provides pointers for developers
About two years ago, Amazon made news when its facial recognition technology falsely scanned and stamped 28 members of congress as convicts or criminals.
Then, Google came under fire for unethically “bribing” people with dark skin tones to be scanned using a phone handed to them, under the impressions that it was a selfie game or a survey.
Since then, consumers or end-users have been very sensitive and cynical about the use of facial recognition technology as a means of access to services or products – a business application that is increasing in use cases.
While the technology has, in some cases, been painted as unethical and unreliable, it does not mean that the capability will stay that way. Like many other digital solutions, they will evolve, improve, mature and eventually be developed without flaw.
Despite the concerns, facial recognition can have a lot of beneficial application, whether that’s in biometric security, or improving customer experience with quick, seamless and contactless ID verification or payments.
To help organizations and AI architects develop facial recognition capabilities that are systematically ethical and reliable, the World Economic Forum (WEF) has drafted out a framework outlining four key practices that tailor trustworthiness.
The practices, it hopes, can help organizations deliver facial recognition technology that is accountable for the data it collects and is ultimately free of bias.
# 1 | Justifying the need for a facial recognition system
A range of products and solutions are out there, so why use facial recognition?
First off, WEF urges businesses considering facial recognition to build a strong business case built to support the use of the technology, and the collection and processing of highly-sensitive personal data.
Among questions that the business case must address include: What are the limitations that businesses are facing? How would facial recognition help solve the problem? How can end-users benefit from the technology deployment? What end results are the business trying to achieve?
Businesses should use market data analysis and research to support their case.
For instance, many airports and airline companies are quickly leveraging facial recognition systems to verify passengers’ ID at different touch points. In those airports, tickets will no longer be needed to verify passengers’ identity in order to check-in their luggage – security and efficiency is improved, and the passenger has a smoother, quicker journey.
# 2 | Devising a data strategy that matches end-users’ traits
An organization seeking to launch facial recognition must have a clear idea of the facial features and traits that would make up the data that the technology will collect. Having sample subgroups that represent a fair variety of features would help make up datasets that can be used to train the facial recognition model.
In the WEF report, it was stated: “Even when using a pre-trained model, it is important to collect a test dataset, specific to the conditions of use and the characteristics of the end-users, to evaluate the system for unfair biases.”
# 3 | Limiting the risks of biases
To limit biases that the system might project, organizations must first identify the kind of ‘discrimination’ and ‘miscalculated judgments’ that the system might project in the context of the business case.
Making sure that the system accounts for age, gender, race, skin tone and non-standardized facial features are key here. During the training process, it’s very important that the model is tested thoroughly and repeatedly to identify risks of biases.
Some ways to improve is to revise the metrics being used to support facial scans data and recognition capabilities. Using high-quality sensors and feeding it with a rich set of data will also help.
# 4 | Being transparent with end-users
Before the technology is put in place, organizations must make it a point to deliver all the necessary information to achieve a sense of trust. End-users are mostly concerned about having their face captured and stored as data because they do not know how will organizations will really use it, or how secure it will be.
In this case, it is important to maintain transparent. This would mean informing end-users of the role of facial recognition, principles that govern the design and use of the technology – in simple, intelligible terms – and lastly, the policies surrounding the data being collected, used, shared and protected.
READ MORE
- Safer Automation: How Sophic and Firmus Succeeded in Malaysia with MDEC’s Support
- Privilege granted, not gained: Intelligent authorization for enhanced infrastructure productivity
- Low-Code produces the Proof-of-Possibilities
- New Wearables Enable Staff to Work Faster and Safer
- Experts weigh in on Oracle’s departure from adland