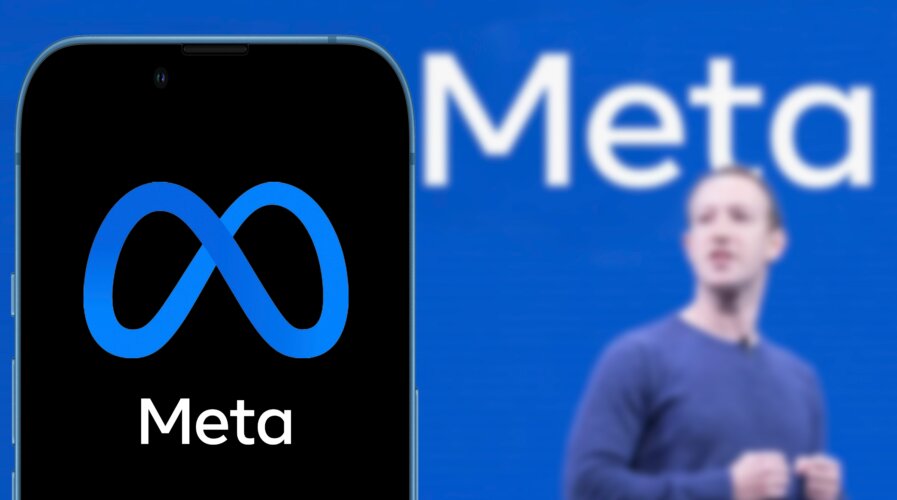
(Source – Shutterstock)
Meta unleashes AI for image segmentation
When it comes to imaging and artificial intelligence, the technology is focused mostly on recognizing a particular image through segmentation. Image segmentation involves computer vision which is when a particular image is divided into multiple segments that represent an object or region in the image,
Image segmentation is primarily the core piece of technology when it comes to object detection. Object detection is what drives image recognition in use cases like autonomous driving, license plate recognition, facial recognition and many more. Object detection can also differentiate between humans, animals, vehicles, texts and even facial expressions.
While this technology continues to evolve and has already been implemented in many use cases today, image segmentation has a lot more to offer in object detection. In fact, creating an accurate segmentation model for specific tasks require more work by experts who have access to AI training infrastructure and large volumes of annotated in-domain data.
As such, Meta has decided to democratize segmentation by introducing the Segment Anything project. The project is a new task, dataset, and model for image segmentation.
“We are releasing both our general Segment Anything Model (SAM) and our Segment Anything 1-Billion mask dataset (SA-1B), the largest ever segmentation dataset, to enable a broad set of applications and foster further research into foundation models for computer vision. We are making the SA-1B dataset available for research purposes and the Segment Anything Model is available under a permissive open license (Apache 2.0),” Meta said in a statement.
Meta aims to build a foundation model for image segmentation: a promptable model that is trained on diverse data and that can adapt to specific tasks, analogous to how prompting is used in natural language processing models. Unlike images, video and texts which are abundant on the internet, the segmentation data needed to train such a model is not readily available online or elsewhere. As such, with Segment Anything, Meta set out to simultaneously develop a general, promptable segmentation model and use it to create a segmentation dataset of unprecedented scale.
“SAM has learned a general notion of what objects are, and it can generate masks for any object in any image or any video, even including objects and image types that it had not encountered during training. SAM is general enough to cover a broad set of use cases and can be used out of the box on new image “domains” — whether underwater photos or cell microscopy — without requiring additional training (a capability often referred to as zero-shot transfer),” Meta explained.
Looking at use cases, Meta believes the SAM could be used to help power applications in numerous domains that require finding and segmenting any object in any image. For example, SAM could be prompted to find specific objects in an image, which can help with image detection use cases.
For the AI research community and others, Meta pointed out that SAM could become a component in larger AI systems for a more general multimodal understanding of the world, for example, understanding both the visual and text content of a webpage. In the AR/VR domain, SAM could enable selecting an object based on a user’s gaze and then “lifting” it into 3D.
For content creators, Meta highlighted that SAM can improve creative applications such as extracting image regions for collages or video editing. SAM could also be used to aid the scientific study of natural occurrences on Earth or even in space, for example, by localizing animals or objects to study and track in video.
So how does Meta’s image segmentation tool work?
According to Meta, SAM is a single model that can easily perform both interactive segmentation and automatic segmentation. The model’s promptable interface allows it to be used in flexible ways that make a wide range of segmentation tasks possible simply by engineering the right prompt for the model (clicks, boxes, text, and so on).
Meta also explained that SAM is trained on a diverse, high-quality dataset of over 1 billion masks, which enables it to generalize to new types of objects and images beyond what it observed during training. This ability to generalize means that by and large, practitioners will no longer need to collect their own segmentation data and fine-tune a model for their use case.
“Taken together, these capabilities enable SAM to generalize both to new tasks and to new domains. This flexibility is the first of its kind for image segmentation,” Meta stated.
The SAM model and dataset will be available for download under a non-commercial license. Users uploading their own images to an accompanying prototype likewise must agree to use it only for research purposes.
READ MORE
- 3 Steps to Successfully Automate Copilot for Microsoft 365 Implementation
- Trustworthy AI – the Promise of Enterprise-Friendly Generative Machine Learning with Dell and NVIDIA
- Strategies for Democratizing GenAI
- The criticality of endpoint management in cybersecurity and operations
- Ethical AI: The renewed importance of safeguarding data and customer privacy in Generative AI applications