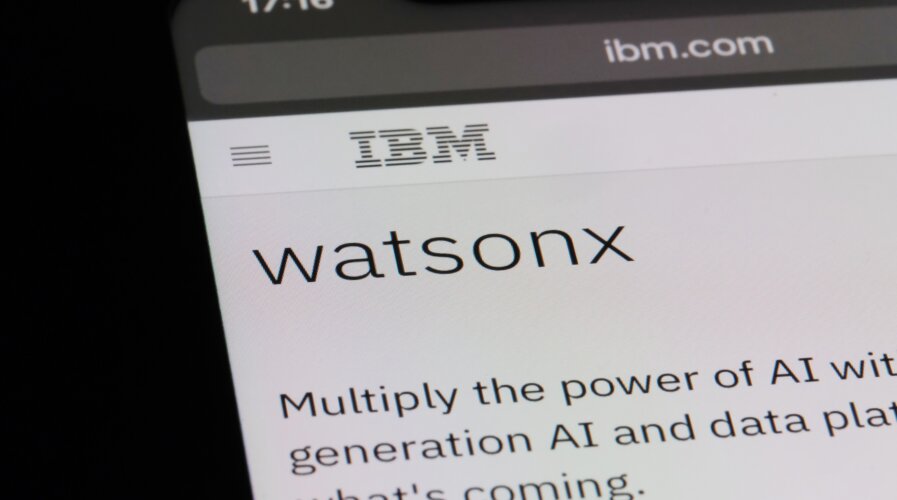
IBM Watson X is a new AI and data platform that will enable enterprises to scale and accelerate the impact of the most advanced AI with trusted data. (Source – Shutterstock)
IBM plans to make a difference in AI with Watson X
ChatGPT is the buzzword for 2023. Almost every organization wants to be involved with this revolutionary technology. The issue is, use cases for ChatGPT are consumer-focused and not useful to enterprises.
While some businesses are using ChatGPT to get work done, the reality is that ChatGPT is only touching the surface of what generative AI and natural language processing can actually do for businesses. In fact, according to a poll by Gartner, more businesses are willing to invest in ChatGPT just based on the hype the technology has generated over the last few months.
According to a McKinsey study, five years ago, 40 percent of respondents at organizations using AI reported more than 5 percent of their digital budgets went to AI, whereas now more than half of respondents report that level of investment. AI adoption is only increasing — with nearly US$16 trillion in investments expected by 2030. In an upcoming study from IBM found 41% of IT professionals say that their company is currently exploring generative AI and 27% are actively using it.
For Kitman Cheung, Data and AI Technology leader at IBM Asia Pacific, there is actually a lot more that generative AI can offer for businesses. As popular as ChatGPT may have become, he believes it is probably not the most appropriate tool for business, which needs a combination of security, scalability, accuracy, trust, and governance.
In a conversation with Tech Wire Asia, Cheung shared how IBM is hoping to make a difference in AI enterprise use cases with IBM Watson X, a new AI and data platform that will enable enterprises to scale and accelerate the impact of the most advanced AI with trusted data.
TWA: How big an impact does AI have on businesses today?
I think we are at that turning point where AI is going to bring in a whole new era of creativity, and innovations. We’re going to find new art, music and creations that we’ve never seen before or probably wouldn’t even think is relevant or possible. From a business perspective, the leading thinkers in every industry are already seeing that potential and how it can be applied.
In Asia, it is a very dynamic market. Many startups are dabbling deep in the area of AI. Chat GPT’s Netscape moment is a big wake-up call for some of the larger, perhaps more traditional organizations to turn around and say – Hey, I got to jump in on this and get going. And, hopefully, IBM will be part of that consideration in partnership so we can create some innovation together.
Introducing the technology behind https://t.co/wQMxpHYzge, IBM’s enterprise-ready AI and data platform to multiply the impact of AI across your business.
Meet the AI and data platform that’s ready for business: https://t.co/hwauWORFaa #Think2023 pic.twitter.com/6RSDWyc7Md
— IBM Research (@IBMResearch) May 9, 2023
TWA: How has the adoption of AI been for businesses working with IBM?
We saw a huge uptake in terms of chatbot use cases throughout the COVID-19 timeframe, just to help with information retrieval and such. The foundation model and large language model (LLM) use cases will continue to drive and increase the uptake of AI use cases.
There are other projects as well that are more research-oriented and rely on machine learning models. I think what’s exciting is that we are also bringing additional foundation models into AI like geospatial. Some of the work that’s being done around the world is being packaged up so that other industries in Asia can start leveraging those capabilities.
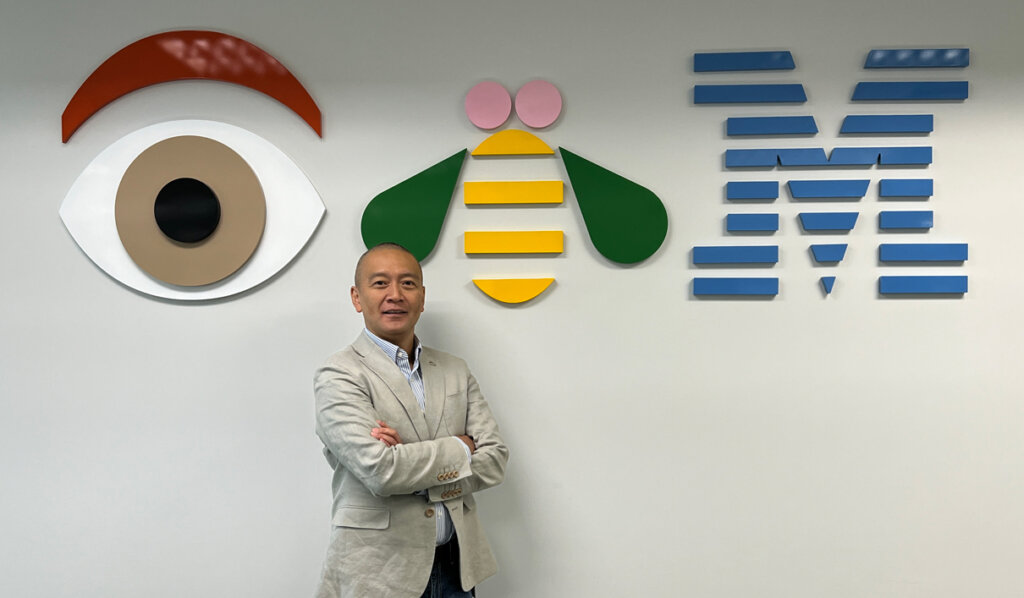
Kitman Cheung, Data and AI Technology leader at IBM Asia Pacific.(Source – IBM)
TWA: What differentiates IBM Watson X from ChatGPT?
We don’t really believe that GPT is going towards a business-oriented AI circumstance. It is more of a consumer tool that drives a lot of interest and strong entertainment. Conversational AI driving entertainment is really important. And I do not mean this as a negative statement. Some of the most compute-intensive use cases for compute are for entertainment like gaming and such.
They do drive some of the leading-edge technology in this space, but I don’t think in reality any business will be able to leverage ChatGPT in its current form to deliver a business outcome because of the hallucination problems that it can have. For example, the provenance of data you used to train the model itself, I think there are a number of limitation factors and it wasn’t designed to solve business problems.
With IBM Watson X, what we’re trying to do is bring the foundation. This means having LLM models and other types of models to train in a way that can be used to solve serious business problems. We want to be able to be guaranteed that the speech that is generated is free of questionable content and that the data used to train that model has the correct provenance – so that you can trust that information. And then, as we extend that model and answer questions for our customers, they can extend their model to answer questions and generate texts. They can be sure that the text generated is based on accurate information about that business.
I think that’s a key element and key differentiation. And in a way, it also makes a model smaller because you are restricting the domain space. In short, we really not going after that consumer part of the space. We’re really looking at, how we build practical, cost-efficient, scalable models so that you can trust your business interaction with the client. I think that’s the space we are going to operate with IBM Watson X.
TWA: How is IBM Watson X more cost-effective for businesses?
IBM Watson X, as a platform, is designed to reduce the barrier of entry in terms of getting to use this type of model and technology. Foundation models take a huge amount of unlabeled data and a lot of facilities to train. Most midsize businesses don’t have the resources, nor would they want to invest in the resources to create their own foundation model, for example.
Foundation models are pre-trained on massive amounts of unlabeled data, which is less expensive to prepare, cutting costs. Once trained, these models can easily be adapted to use cases using 10 to 100 times less labeled data than previous approaches. While foundation models do require significant up-front investments, you get far more than you give because they build on their initial work each time they are used. IBM’s early client engagements are experiencing a 70% faster time to value.
Part of the strategy with IBM Watson X is that while these foundation models are expensive to pre-train up front, let us do that pre-training for you. Instead of having to train your own foundation model, you simply use a small amount of maybe 10% of labeled data to take that last mile of tuning and customization to complete your use case. And you can do that repeatedly.
Some of the early adopters with some of the foundation models that we have are already seeing significant savings in terms of costs of bringing AI solutions. We’re talking about reducing the resolution by 60 to 70% in terms of effort.
It’s a multi-prong approach. Apart from training more people and bringing consultants that can help customers adopt, we are also going to lower the barrier of entry with tools and platforms like IBM Watson X. That’s how are we going to bring together AI and allow more businesses, especially midsize businesses to take advantage of it.
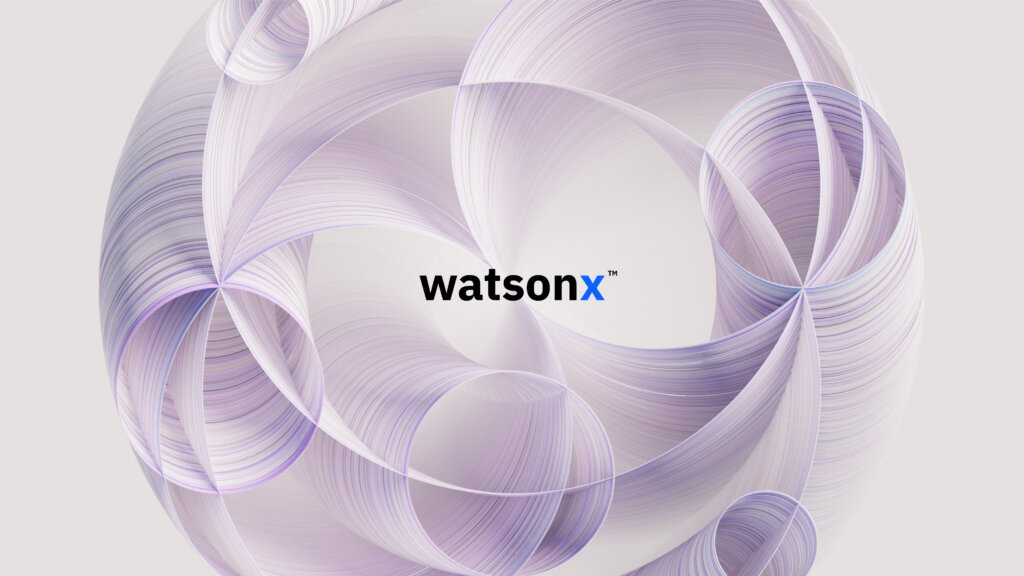
IBM Watson X (Source – IBM)
TWA: What are your views on the growing privacy concerns brought about by AI?
I think there’s certainly legitimate concern whenever any advance and significant events in technology come in, especially for one that’s going to have larger implications. The use case and deciding where to use AI are important, especially on what is permissible. It’s a big responsibility that businesses and individuals really need to take seriously. IBM is actively participating in this area of work and we still believe that government regulation still is currently the most effective way in dealing with this type of AI ethics situation.
As a company, IBM has been calling for a risk-based approach to regulation for several years and have seen that incorporated into AI regulation (NIST framework, EU AI Act), but the rise of foundation models and generative AI is bringing a wave of proposed rules that are not risk-based. IBM recently made four concrete recommendations to the US Congress and policymakers all over the world for how to address AI concerns in a way that recognizes a risk and context-based approach to AI regulation remains the most effective strategy to minimize the risks of all AI – including foundation models and generative AI:
- Promote transparency into the models and their performance
- Leverage flexible approaches
- Differentiate between AI business models
- Look to the future and emerging risks
There is no question that we need to look at that very seriously. The key thing that we are advocating is to look at AI from the angle of use case in context, as opposed to algorithm and technology. I think the pragmatic and practical way of dealing with these issues isn’t to say, “Oh, I will ban all generative AI algorithms”.
Policymakers can address concerns in a way that recognizes that a risk and context-based approach to AI regulation remains the most effective strategy to minimize the risks of all AI, including those posed by foundation models. IBM’s position is to advocate understanding the use case and the context and then putting in the right safeguard and a guardrail to protect against misuse. And I think, to some extent, in the future, AI will also play a role in detecting bad actors as well.
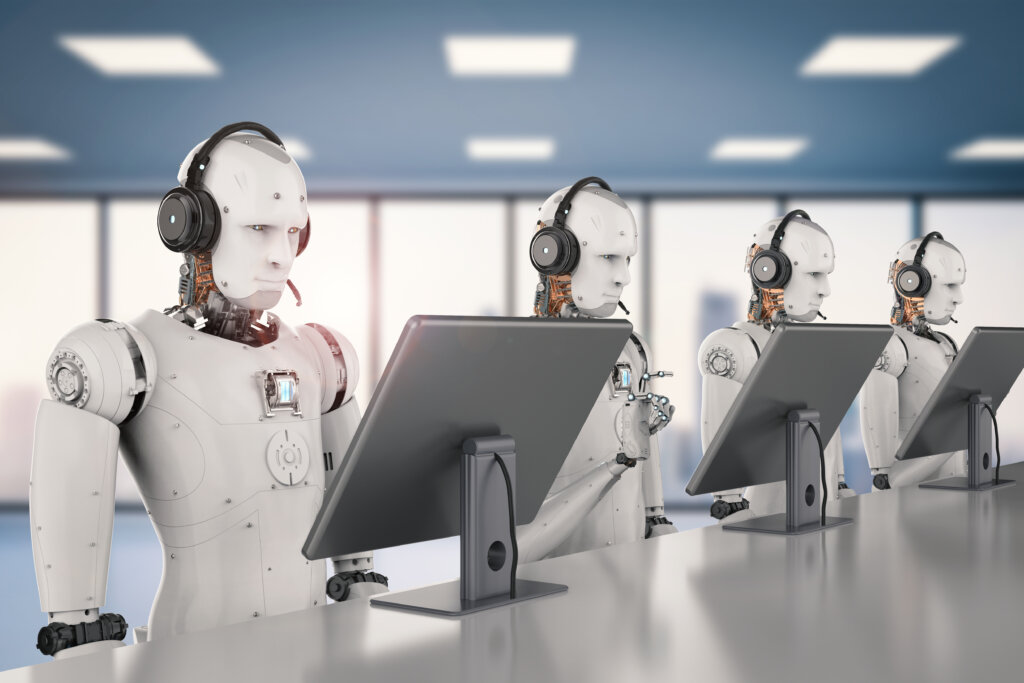
AI will most likely replace some roles but its main purpose is to enhance the workforce. (Source – Shutterstock)
TWA: Apart from privacy concerns, the other big worry is that AI will eventually replace and take over a lot more jobs. What are your views on this?
That’s definitely possible and it is happening to some degree as some organizations are using AI and various types of automation tools to replace, for example, repetitive tasks.
However, it may not be the case for all roles. For example in a call center, technology is not necessarily replacing the call center agent, but replacing some of the simpler, repeating tasks, freeing up the agent to face clients for other matters.
I think the AI use case differentiation is the actual nature of the criticality of that use case like a simpler task, where perhaps an AI can have the same level of interaction as a human can do in an accurate manner.
But when it comes to tasks of a more critical nature, where the decision needs to be made, it is going to be a human task until we’ve sorted out a number of technical and trust issues. When it comes to some critical decision point in any business process, it is very much important to have the AI serves as a companion or a helper to a human employee.
There are some use cases in higher-level tasks we’re already seeing. It may not be generative AI but there are use cases whereby AI in visual detection can help experts in detecting particular problems like medical imaging and so on. That is an augmentation to enhance the way that humans can do their job.
It comes back to the same thing – AI to augment humans to increase productivity to improve the outcome. That’s where AI is most powerful. In some cases, it may actually take over a majority of the workflow. In other cases, it is going to play a much more minor role. We are going to see that change and as it gets smarter, it may take over a portion of it.
READ MORE
- Strategies for Democratizing GenAI
- The criticality of endpoint management in cybersecurity and operations
- Ethical AI: The renewed importance of safeguarding data and customer privacy in Generative AI applications
- How Japan balances AI-driven opportunities with cybersecurity needs
- Deploying SASE: Benchmarking your approach