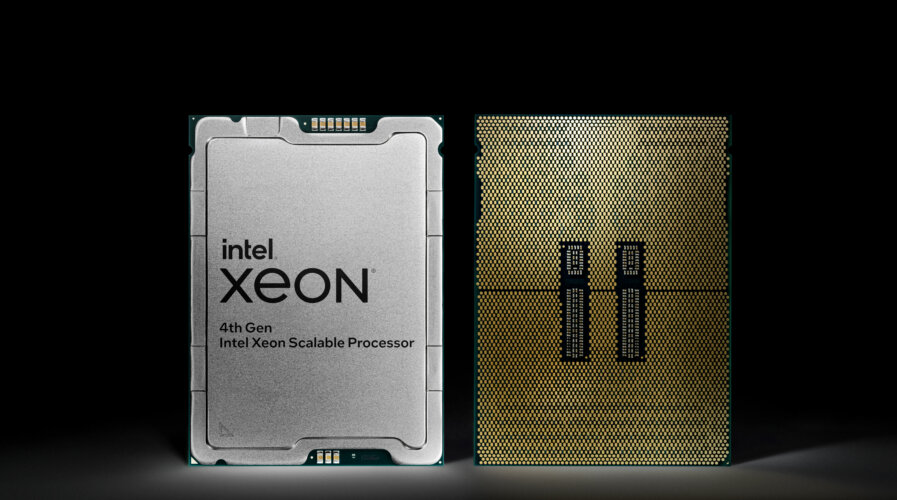
On Jan. 10, 2023, Intel introduced 4th Gen Intel Xeon Scalable processors, delivering the most built-in accelerators of any central processing unit in the world. (Credit: Intel Corporation)
Intel updates its mission to empower generative AI
Everyone’s excited about artificial intelligence (AI) thanks to ChatGPT. But while Open AI’s generative AI makes headlines around the world, many need to understand that ChatGPT only represents a small part of what generative AI is truly capable of doing.
In fact, generative AI can do a lot more than mimic human-generated content. There is now a range of use cases that are leveraging generative AI to improve and enhance productivity. This new wave of AI has also propelled businesses to rush to boost their AI capabilities or figure out in what way can they capitalize on it.
However, what’s not often discussed is the complexity of the computing required to successfully deploy AI. From consumer electronics to the edge and cloud, computing demand will only soar as AI takes off.
This is evident as more tech companies are working towards building chips and solutions that can support the growing need for stronger compute. For example, Intel, NVIDIA and AMD continue to compete to design chips and hardware that is capable of meeting the increasing compute demand by AI use cases.
Tech Wire Asia caught up with Alexis Crowell, General Manager, Technology Solutions, Software & Services, and Vice President, Sales Marketing & Communications Group, Asia Pacific Japan, Intel Corporation to get her views on the latest Intel updates and how the company is hoping to generate the right tools to meet this demand.
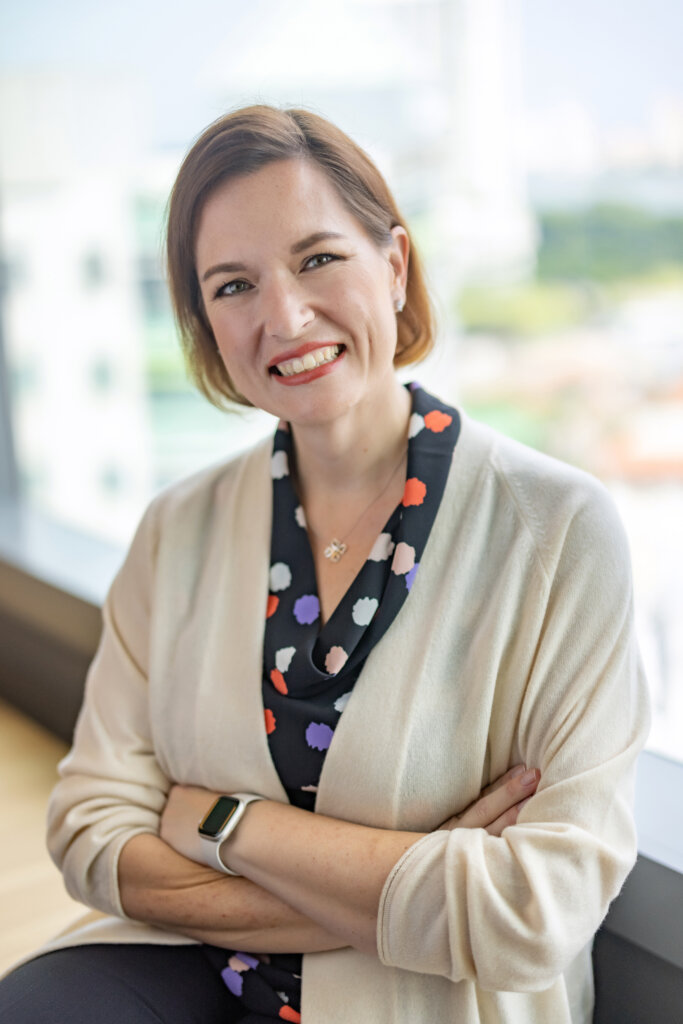
Alexis Crowell, General Manager, Technology Solutions, Software & Services, and Vice President, Sales Marketing & Communications Group, Asia Pacific Japan, Intel Corporation.
TWA: What is Intel’s view on AI and how much of the technology will Intel leverage on its own business processes?
We believe AI is a core tool and functionality that will underpin nearly every type of technology in the future. The industry at one point talked about connectivity and having access to the internet everywhere, and now connectivity is expected. We don’t talk about it as much – the type of connectivity continues to evolve, but just having access, no question. AI is pretty much the same – it will be so pervasive that it will be augmenting and helping everything we do in the future. The types will evolve, and the approaches will change, but the tool itself, we believe, is here to stay.
In addition to providing the computing foundation of hardware and software for AI, Intel as a business is also leveraging AI to enhance our own business processes from finance, HR to Customer Relationship Management (CRM). Intel’s Design Engineering Group (DEG), for example, uses AI and machine learning during power performance tuning to understand what workloads are running (e.g., gaming workload, background task) to then optimize for the specific workload, as well as using AI during validation testing, specifically in pre-silicon validation to minimize post-silicon bugs.
TWA: As AI becomes more powerful and the demand for it soars, the “brains” or chips must also boost their capabilities to accelerate the performance and speed required. What kind of computing platform is needed for AI? How is Intel supporting this demand?
AI is the fastest-growing compute workload from the data center to mobile to the intelligent edge. It has fueled a new level of engagement that will require new levels and specific kinds of compute – chips that will address compute demand but do it in a way that will address performance-per-watt or performance per dollar of ownership expense requirements. As a result, powering AI’s proliferation requires a holistic and heterogenous architecture approach that accounts for vastly different use cases, workloads, power requirements and more. That is why Intel’s silicon portfolio is comprised of a diverse mix of power levels, performance levels, and optimized use cases across CPUs, GPUs, FPGAs and ASICs to support AI’s need for heterogeneous compute.
There is no one-size-fits-all when it comes to computing. Sometimes, general-purpose CPUs are more than powerful enough to support machine learning, or even certain training types for Deep Learning, and in those instances, a GPU would be overkill. Most customers aren’t training from scratch so fine-tuning a model in a matter of minutes is well within the capability of CPUs like the 4th Gen Intel Xeon Scalable Processors.
These processors which with built-in accelerators like Intel Advanced Matrix Extensions (AMX), can achieve 10 times improvement in inference and training performance across a broad suite of AI workloads, while also enabling up to 14 times performance-per-watt increases over Intel’s previous generation.
However, GPUs and CPUs are meant to complement, rather than compete in this regard. Only when it makes sense from a performance, cost and energy efficiency perspective, and the computing platform is flexible and scalable for the changing workload requirements, can we reach AI practicality.
We talk a lot about chips, yet AI is a software problem. You also need a software ecosystem so customers can code in Python (for example) and get the best performance out-of-box. Developers are the subject matter experts, and they want to get the best performance out of the box while remaining as hardware-agnostic as possible. This is where the Xeon software ecosystem like oneAPI and OpenVINO have strength – it is one of the most open, adopted ecosystems out there which allow developers to run their programs on CPUs, GPUs, and FPGAs with minimal code tweaks without constraint. Without an open approach, adoption will be limited.
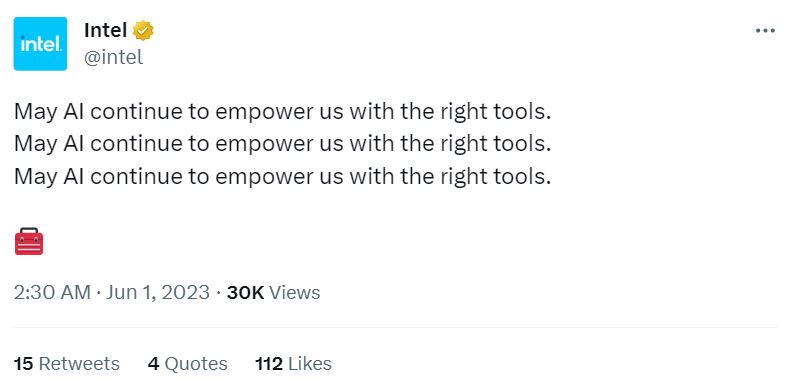
Intel wants to empower AI compute with the right tools.
TWA: Many businesses and individuals want to capitalize on the power of AI. However, just how can we democratize AI and make it as accessible as possible?
The AI workload is complex, made up of structured and unstructured data types and multi-modal models, and costs are an important consideration. Training large models can cost between US$3 to US$10 million for a single model, though we believe the future will be deploying small/medium-sized models, running on general-purpose CPUs.
To achieve the democratization of AI, Intel wants to foster an open AI software ecosystem – driving software optimizations upstream into AI/Machine Learning frameworks to promote programmability, portability, and ecosystem adoption. As an example of the power of open for artificial intelligence: Huggingface is an open language model that can run at nearly the same speed and same accuracy as ChatGPT, but instead of running on a sizable GPU-cluster, Huggingface can run on a single-socket Xeon server. The innovation that can happen in the open is tremendous.
Second, providing choice and compatibility across architectures, vendors, and cloud platforms in support of an open accelerated computing ecosystem. Third, delivering trusted platforms and solutions to secure diverse AI workloads in the data center and inference at the edge with confidential computing. Intel SGX is one of the main technologies powering confidential computing today, enabling cloud use cases that are beneficial for organizations that handle sensitive data on a regular basis. For example, Intel & Boston Consulting Group collaboration enables generative AI using end-to-end Intel AI hardware and software, bringing fully custom and proprietary solutions to enterprise clients while keeping private data in the isolation of their trusted environments.
And finally, scaling our latest and greatest accelerated hardware and software by offering early access testing and validation in the Intel Developer Cloud.
TWA: Demand for more compute power means increasing use of energy as well. How can we maximize compute sustainably?
Our vision is to deliver sustainable computing for a sustainable future focusing on the design of our products, reducing Intel’s carbon footprint from manufacturing, and collaborating with the ecosystem to create standards and scalable solutions for sustainability.
Products like the 4th Gen Intel Xeon processors are our most sustainable data center processors ever delivered. This includes Perf/watt improvements from the most built-in accelerators ever offered in an Intel processor as well as a new optimized power mode delivering up to 20% power savings with negligible performance impact on select workloads. The processors also have built-in advanced telemetry enabling monitoring and control of electricity consumption and carbon emissions and manufactured with 90-100% renewable electricity.
Intel also initiated the Open IP Immersion Cooling solutions and reference design, collaborating with ecosystem partners to meet the data center and industry needs for scalability, sustainability and customization. Intel’s Open IP Immersion Cooling Community includes: Inventec, Foxconn Industrial Internet, Compal, Supermicro, AIC, Acer, 3M, SK Hynix, and much more.
And like I mentioned, the more we foster open environments, the more the ecosystem can iterate and innovate to bring more sustainable solutions to market.
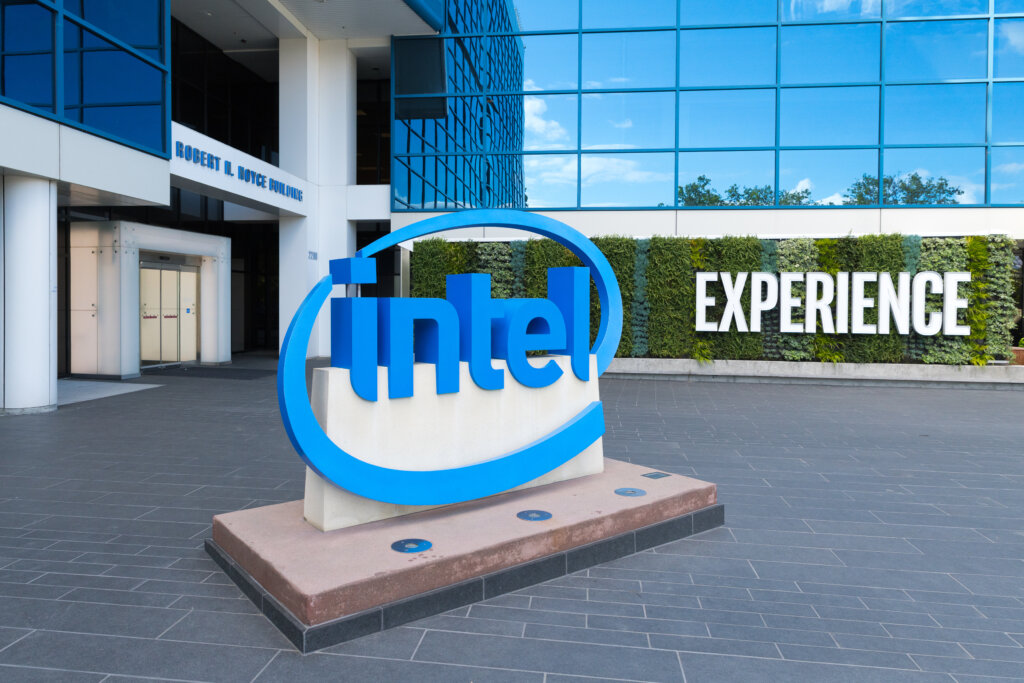
The AI workload is complex and Intel is hoping to provide the right tools for businesses to simplify their AI journey. (Source – Shutterstock)
TWA: Lastly, regulations in AI are being prioritized by most governments currently. What would Intel like to see come out of these regulations on AI?
Some six years ago I helped author our policy on how to approach facial recognition in our technology – to help ensure we are being responsible in what we enable with our customers. This shows our commitment to developing the tech responsibly. Furthermore, we have rigorous, multidisciplinary review processes throughout the development lifecycle, establishing diverse development teams to reduce biases, and collaborating with industry partners to mitigate potentially harmful uses of AI.
We are committed to implementing leading processes founded on international standards and industry best practices. AI has come a long way but there is still so much more to be discovered, as technology evolves. We are continuously finding ways to use this technology to drive positive change and better mitigate risks. We continue to collaborate with academia and industry partners to advance research in this area while also evolving our platforms to make responsible AI solutions computationally tractable and efficient.
Intel is also working closely with governments around the world to share our experiences on areas for responsible AI such as:
- Internal and External Governance: Internally, our multidisciplinary advisory councils review various development activities through the lens of six key areas: human rights; human oversight; explainable use of AI; security, safety, and reliability; personal privacy; and equity and inclusion.
- Research and Collaboration: We collaborate with academic partners across the world to conduct research in privacy, security, human/AI collaboration, trust in media, AI sustainability, explainability and transparency.
- Products and Solutions: We develop platforms and solutions to make responsible AI computationally tractable (such as Intel’s Real-Time Deepfake Detector in video below). We create software tools to ease the burden of responsible AI development and explore different algorithmic approaches to improve privacy, security and transparency and to reduce bias.
- Inclusive AI: We understand the need for equity, inclusion and cultural sensitivity in the development and deployment of AI. We strive to ensure that the teams working on these technologies are diverse and inclusive.
READ MORE
- Strategies for Democratizing GenAI
- The criticality of endpoint management in cybersecurity and operations
- Ethical AI: The renewed importance of safeguarding data and customer privacy in Generative AI applications
- How Japan balances AI-driven opportunities with cybersecurity needs
- Deploying SASE: Benchmarking your approach