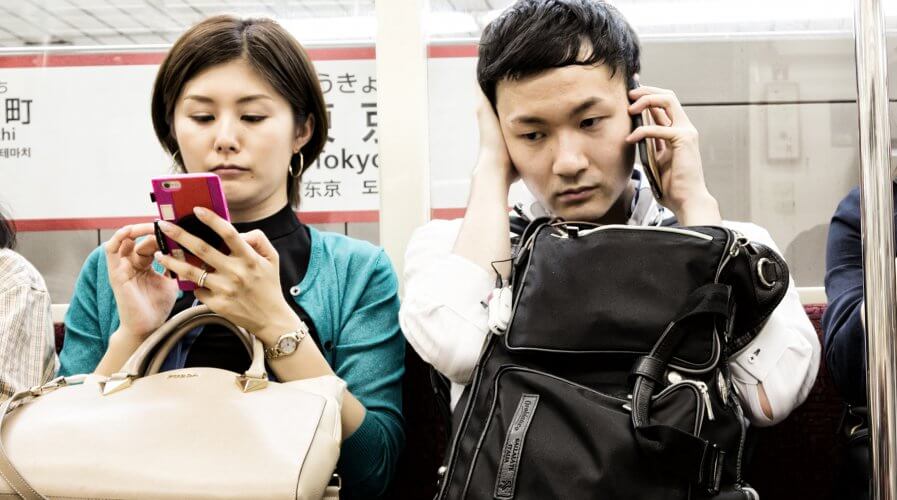
Customers keep all the data, scientists get all the insights, thanks to federated learning. Source: Shutterstock
Google Distinguished Scientist explains what federated learning is
FEDERATED learning is a concept that excites programmers and developers.
However, the term has recently intrigued business leaders because it provides an alternative to collecting huge amounts of customer data to train machine learning (ML) algorithms.
On the surface, it seems as though federated learning makes it easy for organizations to avoid putting customer data at risk without affecting access to the valuable insights that the data brings in the first place — and that’s a big win for organizations looking to make progress with ML without increasing cybersecurity and privacy concerns.
To better understand federated learning, Tech Wire Asia joined a media delegation speaking to Google Distinguished Scientist Blaise Aguëra y Arcas about the topic.
Arcas, who is working on creating awareness about federated learning and providing support and resources to developers using the framework provided, among other things at Google, has some interesting insights to share.
“Federated learning allows Google’s apps and services to work better for you—and work better for everyone—without collecting raw data from your device.
“Instead of sending data to the cloud, we ship machine learning models directly to your device. Each phone computes an update to the global model, and only those updates — and not the data — are securely uploaded and aggregated in large batches to improve the global model. Then the updated global model is sent back to everyone’s devices.
“We are challenging the assumption that products need more data to be helpful.”
Arcas said that Google is currently using federated learning in the Google Keyboard “Gboard” on Android.
Here’s how federated learning works on Gboard:
The device downloads the machine learning model, updates it on the device itself based on things users across the world type, and then uploads the updated model back to the server, without the user’s data ever leaving the device.
After thousands of people begin typing these new words — without Google ever collecting data on what someone types — the tech giant is able to identify them, understand them, and suggest them to users.
What Google does with federated learning on Gboard can easily (theoretically speaking) be replicated by other companies to train their apps without risking the privacy or security of data.
Arcas pointed out that many of the largest successes in ML have come from data that is openly available on the web, such as translated text that many translation services (including Google Translate) have trained on.
In addition, Google AI has released dozens of datasets for ML researchers, from annotated text to images to videos and more to help with training and their ML models.
However, Arcas and his team are excited about the potential of on-device ML and are actively exploring to bring federated learning to more apps and services.
Although conversations about federated learning have picked up only recently, the concept is something that Google Chief Privacy Officer Keith Enright spoke to Tech Wire Asia about earlier this year — and is in line with the company’s overall vision to make data privacy easier and more effective.
Of course, federated learning is also a core part of the Privacy Sandbox that Google recently announced.
In the coming months, both developers and business leaders will hear more about federated learning. To stay ahead of the curve, data scientists in organizations of all shapes and sizes need to start exploring the concept now.
READ MORE
- Safer Automation: How Sophic and Firmus Succeeded in Malaysia with MDEC’s Support
- Privilege granted, not gained: Intelligent authorization for enhanced infrastructure productivity
- Low-Code produces the Proof-of-Possibilities
- New Wearables Enable Staff to Work Faster and Safer
- Experts weigh in on Oracle’s departure from adland