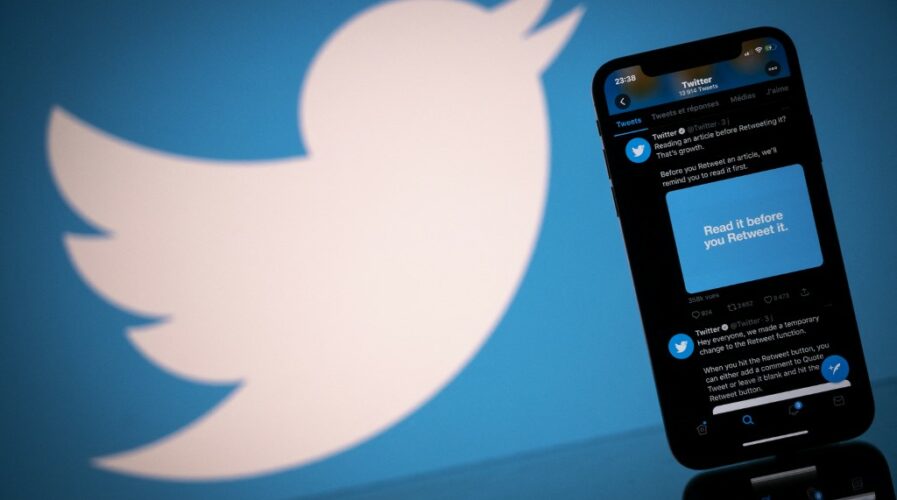
Twitter said it would offer a cash “bounty” to users and researchers to help root out algorithmic bias on the social media platform. (Photo by Lionel BONAVENTURE / AFP)
Twitter ramping up efforts to stomp out misinformation
It seems that Twitter is really making some waves in the AI scene these days. Days after their announcement of an innovative bug bounty challenge to weed out AI bias, the social media giant has shared that they’re working to further put pressure on stamping out misinformation.
And this time, they’re collaborating with The Associated Press (AP) and Reuters. This initiative is not just to push down further on misinformation, but to better identify and prominently highlight credible information posted on Twitter.
According to a release on their website, the company said that they “will be able to expand the scale and increase the speed of our efforts to provide timely, authoritative context across the wide range of global topics and conversations that happen on Twitter every day.”
Currently, Twitter surfaces credible information and context through a Curation team to help readers make informed decisions about what they see on Twitter.
When large or rapidly growing conversations happen on Twitter that may be noteworthy, controversial, sensitive, or may contain potentially misleading information, Twitter’s Curation team sources and elevates relevant context from reliable sources.
These added context and reliable information can be found in several places on Twitter, such as in the trends section, explore tab, search prompts, and label.
This new collaboration will boost these efforts by increasing their capacity to add reliable contexts to conversations on Twitter, increasing the scale and speed of work currently undertaken.
Partnering with highly reputable, reliable news sources such as AP and Reuters can help inform the Twitter Curation team, who may at that time not have specific expertise or resources to verify the credibility or accuracy of the content.
According to the release, Twitter said that this goes towards “Ensuring that credible information is available in real-time around key conversations as they emerge on Twitter”.
Twitter has a decently long history of using deep learning to surface relevant content on timelines.
Earlier this year, they launched the Responsible Machine Learning initiative, which is designed around four pillars, namely – taking responsibility for algorithmic decisions; equity and fairness of outcomes; transparency about decisions, and the enablement of agency and algorithmic choice.
Twitter realizes that technical solutions alone do not resolve the potentially harmful effects of algorithmic decisions, which is why their Responsible ML working group is interdisciplinary and is made up of people from across the company, including technical, research, trust and safety, and product teams.
Leading this work is their ML Ethics, Transparency, and Accountability (META) team: a dedicated group of engineers, researchers, and data scientists collaborating across the company to assess downstream or current unintentional harms in the algorithms used and to help Twitter prioritize which issues to tackle first.
Twitter said last week that it would offer a cash “bounty” to users and researchers to help root out algorithmic AI biases on the social media platform.
It would be “the industry’s first algorithmic bias bounty competition,” with prizes to the tune of US$3,500.
In the months or years to come, we expect to see a more nuanced timeline that’s contextually more relevant and accurate, which is expected to cut down on misinformation, especially in countries like India where it is rife.
It augurs well for the future when tech giants like Twitter take heed of and embark on such efforts to be responsible and accountable for the technology they utilize.
Hopefully, this can motivate other tech companies at large to seriously consider more responsible machine learning and deep learning use in their processes, especially when it comes to important segments such as automotive or healthcare, among others.
READ MORE
- Strategies for Democratizing GenAI
- The criticality of endpoint management in cybersecurity and operations
- Ethical AI: The renewed importance of safeguarding data and customer privacy in Generative AI applications
- How Japan balances AI-driven opportunities with cybersecurity needs
- Deploying SASE: Benchmarking your approach