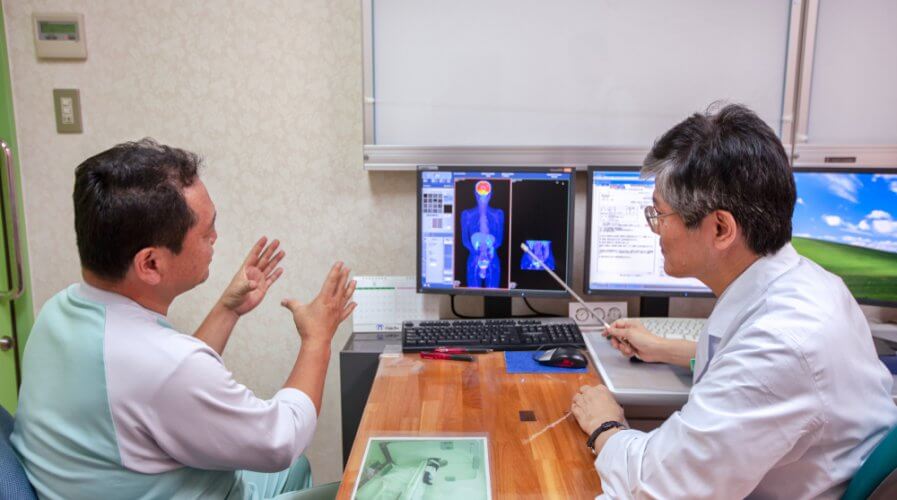
Artificial intelligence has made a debut in a number of industries, but are we making the most of the technology? Source: Shutterstock
Could a failure to scale artificial intelligence projects spell doom in 2020?
THERE IS no technology as powerful as artificial intelligence (AI), that much is certain.
In the world of business, organizations have shown faith in the technology by investing in a number of pilot projects — but unfortunately, they have been unable to scale up those projects to unleash the full potential of AI.
According to a recent survey of 1,500 c-suite executives, 75 percent said they believe they risk going out of business in 5 years if they don’t scale AI.
Further, 84 percent of the executives surveyed said they believe they won’t achieve their growth objectives unless they deploy AI.
To top it all, the survey also revealed that the few companies that actually managed to strategically scale AI projects seem to have nearly twice the success rate and three-times the return from AI investments as compared to those pursuing pilot projects in silos.
Given that spending on AI has surged in the past few years, with just the 1,500 companies surveyed reportedly spending about US$306 billion in the last 3 years alone — business leaders need to sit up and think about how they can take AI projects past the pilot phase and scale them up starting next year.
What does it take to strategically scale artificial intelligence projects?
Since the survey was able to spot a few companies that successfully scaled their AI projects — strategically — analysts were able to distill the critical success factors that other companies struggling with AI could consider.
# 1 | Drive “intentional” AI
“Creating value from AI requires leaders to anchor AI in C-suite objectives.”
According to the report, when business leaders champion AI projects that they have a vision for — across the organization — it not only gets piloted and scaled faster but also tends to cost less money.
Nearly three-quarters of those that were successful with AI said they had a clearly-defined strategy and operating model for scaling AI projects in place, while only half of the companies in proof of concept were able to claim the same thing.
In fact, those that scaled successfully also got things like accountability, funding, process definition, flexibility, and other factors right, and did better when compared to those that struggled to scale AI projects.
# 2 | Tune out data noise
“Strategic scalers recognize the importance of managing data.”
Businesses today collect a lot of data. In fact, 90 percent of data in the world was created in just the past decade. With the arrival of 5G and the proliferation of the internet of things (IoT), companies will collect more data than ever before — up to 175 zettabytes of data by 2025 according to a forecast by IDC.
However, in order for businesses to truly leverage AI meaningfully, they need to first be able to separate the data from the noise and feed the right information into their AI models.
According to the study, those that succeeded with scaling AI paid close attention to data and invested heavily in data quality, data management, and data governance frameworks on the cloud. Further, those businesses built clear models for the generation of data as well as the consumption of data.
As a result, 61 percent of those that scaled AI accumulated larger, more accurate data sets as compared to 38 percent of those that didn’t. They were also more likely to integrate both internal and external data sets as a standard practice compared.
# 3 | Treat AI as a team sport
“The effort of scaling calls for embedding multi-disciplinary teams throughout the organization in addition to having sponsorship from the top.”
Scaling AI projects is no easy feat. It’s something that requires a fair amount of dedication, communication, and foresight. As a result, business leaders that scale AI successfully tap into multi-disciplinary teams to help them.
According to the report, 92 percent of AI success stories involved multi-disciplinary teams led by chief AI, data, or analytics officers, and comprised of specialists such as data scientists; data modelers; machine learning, data, and AI engineers; visualization experts; and data quality, training, and communications specialists.
At the end of the day, the reality is that scaling AI projects requires all hands on deck and demands that all employees be on board with the change that is being driven.
Hence, those that reported success with AI projects in the survey were significantly more likely to provide their employees with all the training and support needed to help them with the transition — which included providing them with formal training, explaining how AI will change their role, and offering them a role and responsibilities in the transition.
Given the rapidly changing marketplace and the rise of digital-first challengers/disruptors in every industry, business leaders struggling with scaling AI projects need to get serious about overcoming the challenges in 2020.
Failure will only lead to the business making way for competitors — from the same or even lower weight classes — and cause the business to lose market share and customers.
READ MORE
- Safer Automation: How Sophic and Firmus Succeeded in Malaysia with MDEC’s Support
- Privilege granted, not gained: Intelligent authorization for enhanced infrastructure productivity
- Low-Code produces the Proof-of-Possibilities
- New Wearables Enable Staff to Work Faster and Safer
- Experts weigh in on Oracle’s departure from adland