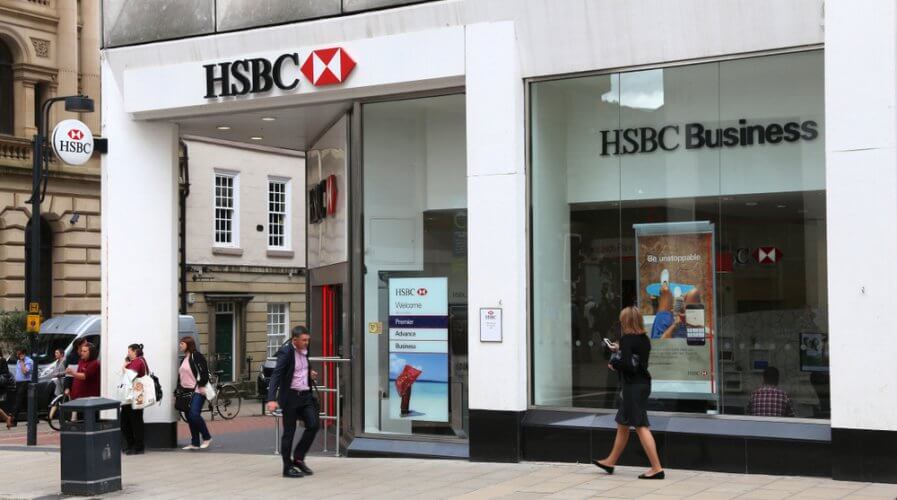
HSBC Bank and others use machine learning to win big. Source: Shutterstock
Here’s why machine learning is critical to success for banks of the future
MACHINE learning is a popular buzzword today, and has been heralded as one of the greatest innovations conceived by man.
A branch of artificial intelligence (AI), machine learning is increasingly embedded in daily life, such as automatic email reply predictions, virtual assistants, and chatbots.
The technology is also expected to revolutionize the world of finance. While it is slower than other industries in embracing the technology, the impact of ML is already visibly significant.
Most recently, HSBC said that the bank was using the technology to combat financial crime.
“We have 608 million transactions every month. Hence, with AI and machine learning we are able to identify a good transaction done by an innocent person versus transaction conducted by criminals,” said HSBC Hong Kong Financial Crime Threat Mitigation Regional Head Paul Jevtovic.
Like HSBC, several other banks are beginning to deploy ML at scale. Here are the top three use cases in the banking and financial services space:
# 1 | Customer-centric solutions
Prior to the advent of ML, decisions were made on a rule-based system, where the same criteria are applied across a broad customer segment, subjecting them to a one-size-fits-all solution.
With ML, bankers can approach customers in a more personalized way. ML algorithms can analyze volumes of consumer data in banks, tracing each customer’s digital footprint with a unified, omni-faceted view.
This footprint includes their financial status across multiple accounts, financial investments, and banking transactions.
With the relevant data and armed with the right analytical tools, ML can provide valuable insights that allow banks to create tailor-made solutions based on a specific customer’s behavior, preferences, and requirements.
# 2 | Risk Management
With the wide tracking of a customer’s digital footprint that ML offers, banks can quickly and accurately assess a potential borrower’s ability to repay — better than with traditional methods.
Leveraging ML can eliminate biases, and can quickly help differentiate between applicants who are more credit-worthy from those who have a higher default risk — even without an elaborate credit history. ML can also help banks forecast potential issues and rectify them before they occur.
With the assurance that risks are being mitigated, banks can focus on issues that can add value to their customers, increase productivity, and provide greater support to their employees.
# 3 | Fraud detection
ML can be greatly leveraged for fraud detection. Fraud is a pain point for many financial institutions, one which could potentially cause a bank to go out of business.
With ML, anomalies in customer’s behaviors can be quickly detected. By flagging and blocking transactions that are suspicious, banks can catch fraud in real-time, protecting customers and themselves.
ML is undoubtedly one of the greatest technological feats of the 21st century. With its laser precision in predicting behaviors and anticipating risks, we can be sure that the role of ML will only be more prominent in the future of banking.
Regardless of size, financial institutions or businesses looking to engage financial services must be aware of the uses of ML in banking. Should they wish to stay relevant, they must start exploring the technology now.
READ MORE
- 3 Steps to Successfully Automate Copilot for Microsoft 365 Implementation
- Trustworthy AI – the Promise of Enterprise-Friendly Generative Machine Learning with Dell and NVIDIA
- Strategies for Democratizing GenAI
- The criticality of endpoint management in cybersecurity and operations
- Ethical AI: The renewed importance of safeguarding data and customer privacy in Generative AI applications