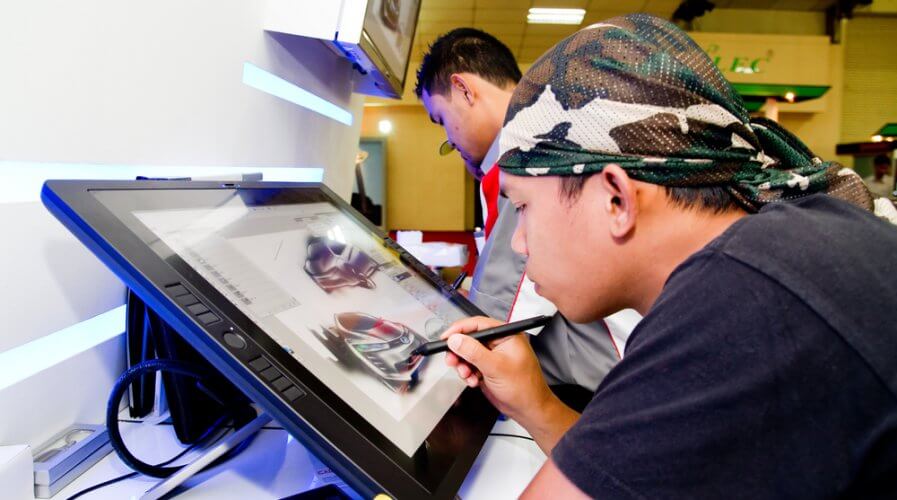
AI and edge computing must go hand in hand. Source: Shutterstock
Let it go: Why AI should move from cloud to edge-computing
AN EXPANDING network of connectivity surrounds us today.
From smart devices that can sense the temperature to connected machines in factories that can ‘talk’ to one another and make decisions autonomously — the number of connections made between humans and devices, or between devices over the internet of things (IoT), is increasing exponentially.
The proliferation of IoT devices brings along with it an avalanche of enterprise data. Having much data is a good thing, but only if meaningful, actionable insights can be derived from it.
To be of use, data collected from each IoT device should not be analyzed in isolation. To derive true value, data sets from multiple devices should be combined to uncover patterns and trends that can fuel predictive analytics.
Artificial intelligence (AI) can do just that. It ‘learns’ from data, and provides accurate, automated feedback to guide decision making. It also produces analyses on how performance can be optimized in various scenarios.
It is no wonder, then, that AI, combined with IoT capabilities, is a force to be reckoned with when it comes to tapping into the full potential of data.
Businesses often turn to the cloud to host their data, which is aggregated in the cloud’s data center where AI-decisions are being made.
However, time for data transfer as well as latency will increase with the distance between the data center and its source. This impedes making real-time decisions. Soon, the cloud will no longer be enough to sustain the increasing demands of IoT applications.
Here’s where edge computing comes in.
AI at the edge can be thought of as a decentralization mechanism of sorts. Instead of having to go to a big processing center (cloud), small clusters of computing devices now work together to drive local decision making.
With edge computing, real-time response is greatly enhanced.
Because there is no need for transferring data to the cloud for processing, latency issues can be eliminated. This will help in improving the validity of real-time decision making.
Further, edge computing enhances risk management, reducing the chance of occurrence of security breaches. Because it is localized, it is easy to implement mitigation tactics. An AI-driven risk analysis can effectively identify any anomalies, and proactively create plans to defend the network.
Also, operational costs can be significantly reduced on the edge as data is processed locally. There is no need for expensive bandwidth and connectivity, allowing resources to be channeled into other business opportunities.
Further, the real-time decision making that edge computing provides also contributes to saving costs. This is because it ensures process uptime by preventing asset breakdowns or sudden failures.
The benefits of AI on the edge is multi-fold and must be acknowledged by enterprises that wish to go digital.
With more IoT devices being deployed, the need for AI-enabled solutions will only grow, and the edge is infrastructure that can best support these operational goals.
Thus, it would be wise for enterprises to look into investing in not just AI-driven solutions, but also migrating these solutions from the cloud to the edge.
READ MORE
- Safer Automation: How Sophic and Firmus Succeeded in Malaysia with MDEC’s Support
- Privilege granted, not gained: Intelligent authorization for enhanced infrastructure productivity
- Low-Code produces the Proof-of-Possibilities
- New Wearables Enable Staff to Work Faster and Safer
- Experts weigh in on Oracle’s departure from adland