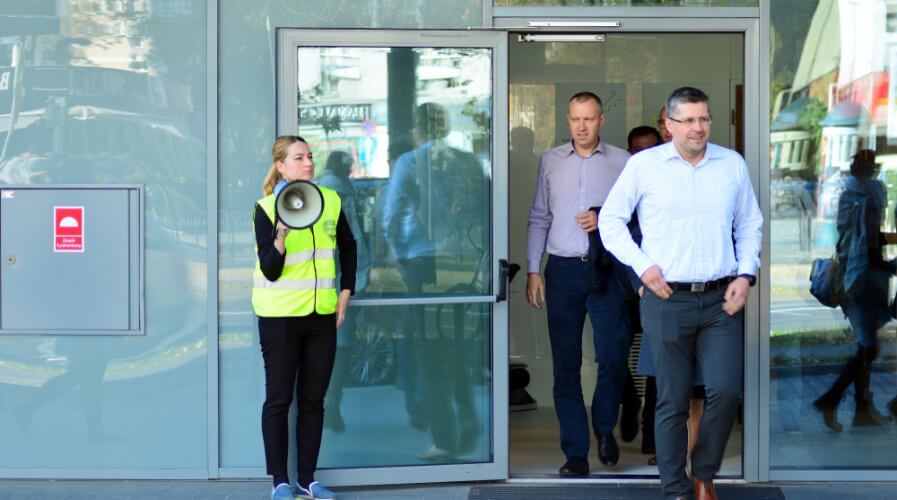
Ethics in AI is critical. Source: Shutterstock
Why subject matter experts must weigh in on AI models
CAPTURING data in today’s world is easy. Be it an action in the digital world on a website or an application or in the physical world in a retail or commercial environment — everything can be tracked. Making sense of that data, however, requires more than just employing data scientists.
Quilt.ai Founder and Chief of Product Angad Chowdhry told Tech Wire Asia that subject matter expertise is the most important piece of the puzzle when it comes to making sense of data.
Chowdhry’s company works with a variety of for-profit and non-for-profit businesses, tapping into data from a plethora of sources, and running artificial intelligence (AI)-powered models to answer questions that help better understand markets, invest resources, and plan for the future.
Quilt.ai recently collaborated with School of Oriental and African Studies (SOAS) and the Barbican Centre in the UK on a project to help AI understand the context when it sees a photograph.
The project involved working with SOAS in taking a list of sub-cultures from the city of London and building a model using user-generated data. This use of “grounded theory”, a sociological concept, in building AI models is because of the subject matter expertise from Quilt.ai (whose founder is an anthropologist by training and equipped to understand the nuances of such data). This culminated in an app that classifies people’s cultural references and signals – that was exhibited at the Barbican
Dubbed Culture.Trace, the project aimed to explore what an alternative vision of a future AI could look like through showing how technology, and the people it analyses, are most interesting when approached through a non-judgemental and culturally empathetic lens. The app also “matched” individuals to other people who had similar cultural DNA across the world. In this experiment, AI was used to “connect” people, not just “classify” them.
“Right now, the issue is not that the machines are biased. The issue is that ground up, culturally sensitive models of AI do not exist. Commercial computer vision models are designed to recognise demographics, age or race when they see images and video, instead of meaning, cultural context, semiotics, etc,”
In a business context, however, there’s an emphasis on bringing subject matter expertise into the fold of data and AI. Too often, organizations recruit data science professionals at scale and give them the data, tools, and resources they need in order to create exciting products and solutions.
Unfortunately, the teams fail to deliver meaningful results within reasonable timeframes since they lack the knowledge-based support and leadership from those with subject matter expertise.
In the restaurant-franchise business, for example, cashless payment options and loyalty programs make it easy to create customer profiles and track their tastes and preferences linked to a particular time of day, location, and menu, among other things.
If only data scientists evaluate the data to create business insights, however, the inability to reach target for white wine sales at noon despite significant sales of white-sauce pastas and chicken dishes might signal that the wine list needs another look.
However, the reality might be that the restaurant might be in a central business district or close to a religious institution or a university, with patrons preferring to avoid alcoholic beverages during working hours. Only the restaurant manager will be able to answer that question.
Similarly, Quilt.ai’s Chowdhry points out that most institutions engaged in the ethics in AI discussion don’t lean on individuals in backgrounds in moral philosophy making it much harder for the world to reach a conclusion than it needs to be.
“There are so many debates around AI and ethics taking place, and I’ve yet to see serious references to Nietzsche or Kant; even modern philosophers are referenced in passing. It would be useful if questions of ethics were not left to just developers, just as questions of Machine Learning Architecture are not left to moral philosophers.”
According to Chowdhry, anyone (especially business leaders) developing AI models and using data to create insights should take note of the fact that solving most problems require subject matter expertise and hence, should involve experts who can provide the context required to make data more meaningful.
In the coming months, as business leapfrog into the era of AI-powered decision-making, the access to subject matter expertise, or lack thereof, will decide what organizations are successful and which ones fail to make a mark.
READ MORE
- Safer Automation: How Sophic and Firmus Succeeded in Malaysia with MDEC’s Support
- Privilege granted, not gained: Intelligent authorization for enhanced infrastructure productivity
- Low-Code produces the Proof-of-Possibilities
- New Wearables Enable Staff to Work Faster and Safer
- Experts weigh in on Oracle’s departure from adland