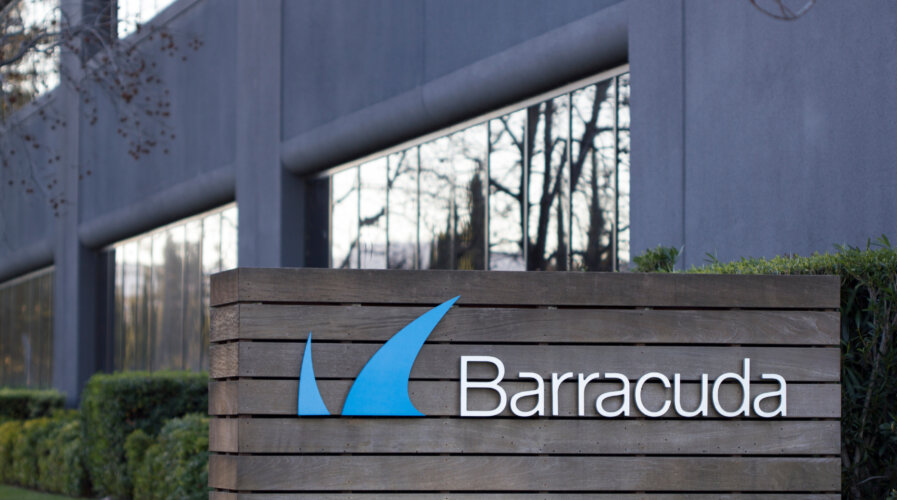
Barracuda’s pioneering vision for generative AI in cybersecurity. (Source – Shutterstock)
Navigating the ethical AI dilemma with Barracuda’s insight on dual-use technologies
- AI technologies, led by OpenAI, are revolutionizing cybersecurity.
- Barracuda’s CTO, Fleming Shi, highlights their AI use, contrasting with OpenAI’s approach.
In the ever-evolving landscape of technology, two elements have come into sharp focus: AI and cybersecurity. The surge of AI-driven applications, from chatbots to content generators, promises vast potential for enterprises. However, the alignment of AI with the intricate world of cybersecurity yields a plethora of both challenges and opportunities. Organizations like OpenAI and Barracuda are making strides in their respective domains.
While OpenAI’s prowess in generative AI models like ChatGPT is widely recognized, Barracuda’s expertise in cybersecurity brings about a different perspective. Continuing from the first part of the interview we did with Barracuda’s CTO, Fleming Shi, we delve into Barracuda’s approach towards integrating AI with cybersecurity and to understand the ethics, challenges, and future of this convergence.
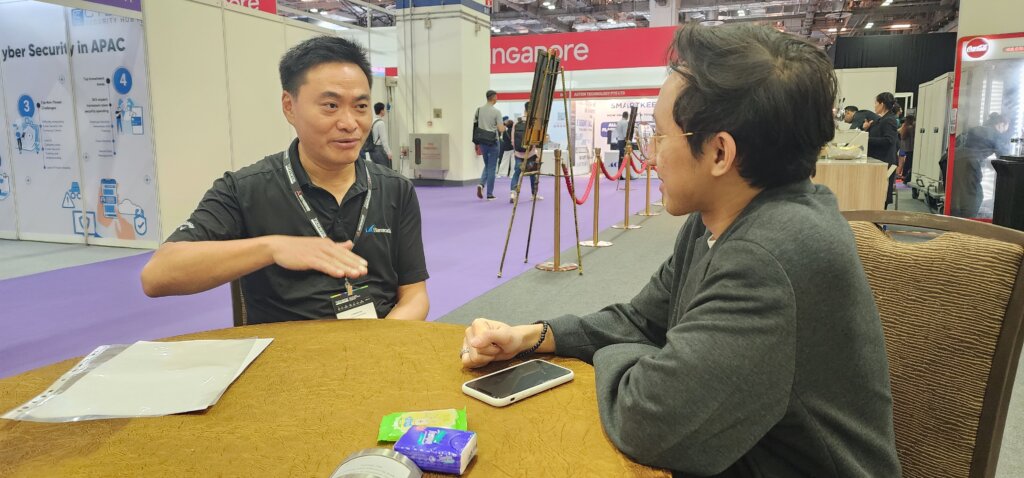
Tech Wire Asia speaks with Barracuda’s CTO, Fleming Shi.
A deep dive into AI technologies: Comparing Barracuda and OpenAI
TWA: With OpenAI’s generative AI, like ChatGPT, the training models were accessible to the general public, allowing the models to learn from user inputs. Does Barracuda have a similar approach, like a “beta model” for users to test, or do you work with a final version internally?
When building a large language model or generative AI tool, the process starts with a foundational large language model. This could be something like GPT 3.5 Turbo, which operates in the same realm as the ChatGPT model but is tailored to operate within a specific environment. The next step involves using a vector database to ingest actual descriptive data.
Comparing Barracuda with OpenAI, it’s evident that the two have different core competencies. Whereas OpenAI primarily focuses on AI, Barracuda’s specialization lies in cybersecurity. Consequently, the data Barracuda gathers, especially from billions of daily emails, substantially differs from the data OpenAI would collect.
We ensure PII protection, and our primary focus revolves around domain perception. We achieve greater accuracy by amalgamating our domain expertise with a vector database and a large language model, especially for cybersecurity tasks.
TWA: How do you ensure the generative AI tools Barracuda uses do not have embedded vulnerabilities, and how do you test these implementations?
While achieving perfection is challenging, we place immense significance on content creation and maintenance. We host a platform called Campus, containing around 22,000 tech documents relevant to the industry. However, some of this documentation, written a decade ago, requires updates.
We continually update the information in our indices, ensuring integration with the large language models. We’re also focusing on making these models modular so we can replace or adjust them as needed. In some instances, we even utilize GPT-4, which is based on 1.7 trillion parameters, for enhanced accuracy over models with 10 to 75 billion parameters. This endeavor is a continuous learning process, and we highly value user feedback. For instance, our tool features a feedback button, allowing users to highlight inaccuracies, helping us to improve.
From an engineering perspective, improvements are constantly underway. However, fine-tuning these extensive language models is not a current focus, primarily due to the high costs. For context, just the hardware for GPT-3 cost about US$5 million, without accounting for electricity and time. In contrast, GPT-4’s hardware costs surged to a hundred million dollars. A fitting analogy would be the frequency with which we might consider ‘retraining’ a widely spoken language like English.
Language models resemble widely spoken languages and, while they evolve with new terms, retraining them entirely is not feasible. Currently, very few companies can undertake this task effectively. Microsoft’s collaboration with OpenAI and AWS’s venture with Anthropic are notable examples.
Ethical implications and safeguarding AI-driven development
TWA: Given generative AI’s dual use in cybersecurity defense and offense, how does Barracuda approach the ethical implications of its development and deployment?
We’re navigating a delicate balance here. Coding assistants have been around for some time. I recall using integrated development environments (IDEs) and generating code 25 years ago. With modern generative AI, one can input a code snippet and a functionality description, and the AI can then adapt that code into various programming languages. This capability is especially beneficial when working on legacy systems – take COBOL, for instance.
COBOL code reads quite like English, with terms like “PERFORM” and “BEGIN.” While most people can comprehend its basics, the real challenge arises when the coding becomes convoluted. This is where tools like generative AI play a pivotal role. They act as guardrails. For instance, if you execute a shell command in a particular programming language, there are multiple ways to write it. Some of these methods might introduce vulnerabilities, such as opportunities for shell injection, potentially compromising the system’s backend.
However, AI-driven tools, trained on vast datasets with vigilant styles, can identify these pitfalls and offer corrections. Enhancements in this area are ongoing.
At Barracuda, while we prioritize the safety of users of products similar to GitHub Copilot, we don’t neglect the security of our developers. Our expertise lies in aiding users of generative AI, especially in SaaS applications, against threats such as phishing attacks. Although we aren’t deeply entrenched in the “shift left” movement, which emphasizes developer-centric security, we recognize the need to secure our coding processes for the benefit of our users.
One significant measure we’re implementing is instilling a “zero trust” approach towards developers. This means mandating the use of co-signing certificates unique to each device. Thus, when code is written and committed, we can ascertain the identity of the coder and the specific device used. Such measures are especially crucial in a software supply chain attack, as they allow us to pinpoint the origin of the compromised code.
Current technological advancements readily support this level of scrutiny. Most devices come equipped with a Trusted Platform Module (TPM) – a secure crypto-processor designed for cryptographic operations. This chip is fortified with multiple physical security features that render it resistant to tampering. Malicious software finds it nearly impossible to interfere with the TPM’s security functionalities.
Essentially, each device, whether a laptop or a phone, possesses a unique “digital DNA.” This concept isn’t new; it’s been around for about 15 years. However, its potential hasn’t been fully leveraged until now. We’re committed to harnessing this technology for enhanced security.
Embracing AI’s rapid development with caution
TWA: In light of calls from industry leaders for an “AI pause,” what are your views on the current pace of AI’s development and deployment in the security sector? Are there new methodologies or tools you’re contemplating?
Pausing AI development seems a bit redundant in today’s landscape. Major players, like Microsoft, have already made their stance clear and are actively pushing AI forward. We must now adopt and integrate AI responsibly.
Drawing a parallel to the evolution of the automobile industry, we can’t neglect safety. Just as the earliest cars may not have had advanced safety features, we can’t just launch AI without ensuring safeguards. Over time, regulations will inevitably emerge, which will then be followed by certifications. These certifications will reassure consumers about the safety of the products they purchase. For example, a Barracuda product with generative AI might be certified to ensure it doesn’t inadvertently expose data.
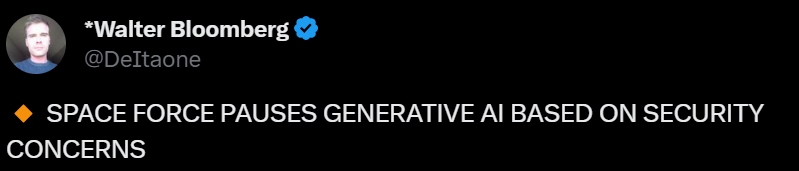
Putting a pause on generative AI. (Source – X)
In essence, certifications will cultivate an ecosystem wherein generative AI can flourish safely. Barracuda aims to be at the forefront of this transformation, especially within the cybersecurity sector. We aim to harness generative AI to aid our clients, as there’s a significant resource deficit in addressing all cybersecurity challenges.
Basically, halting our AI progress impedes innovation and gives our adversaries a potential advantage.
READ MORE
- 3 Steps to Successfully Automate Copilot for Microsoft 365 Implementation
- Trustworthy AI – the Promise of Enterprise-Friendly Generative Machine Learning with Dell and NVIDIA
- Strategies for Democratizing GenAI
- The criticality of endpoint management in cybersecurity and operations
- Ethical AI: The renewed importance of safeguarding data and customer privacy in Generative AI applications